Insuring the weather: modeling the complexities of climate change
Extreme weather makes forecasting and quantifying insurance losses harder, and ‘cat’ models struggle to predict events more extreme than those in the past. As regulators demand more action, dynamic ‘earth system’ models offer a better way to anticipate more climate-change risks.
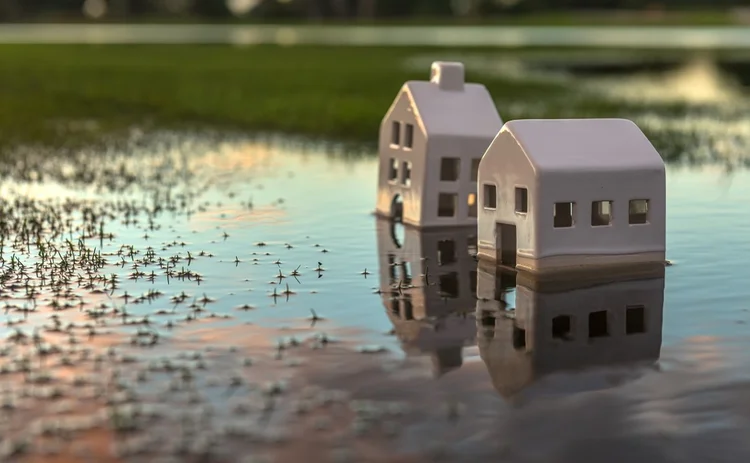
A complex reality
The evidence is overwhelming: we are witnessing more destructive natural disasters caused by climate change. According to the World Meteorological Organization’s 2019 ‘State of the Global Climate Report’, the physical and financial impacts of global warming are accelerating. Carbon dioxide (CO2) levels in the atmosphere were at 257 parts per million (ppm) in 1993, and are now at 407.8 ppm, with further increases expected. The year 2019 concluded a decade of record global temperatures, rising sea levels and melting ice caused by rising levels of greenhouse gases, and these in turn have directly amplified the risk of weather-related disasters. In the past year, every inhabited continent has been struck by extreme weather events including floods, storms, droughts and wildfires.
In 2019, global economic losses related to weather and natural events hit $232 billion, and insured losses reached $71 billion, 6% above the current average for the 21st century. And the full impact of catastrophic events such as the recent wildfires in Australia will not be known for several weeks. So far insurance claims from the fires have exceeded $AUS 700 million, while longer-term costs to the economy are expected to rise as a result of increased air pollution and direct harm to industries such as farming and tourism.
For insurers, the cost of climate change is only likely to increase, because losses related to physical risk factors generate higher claims and directly impact their liabilities. Physical climate risks also disrupt supply chains, increasing production costs and reducing the speed and responsiveness of delivery. So insurers must consider not only first-order risks, but also second- and even third-order impacts.*
To protect themselves, insurers are pushing for premium-rate increases and canceling more policies belonging to customers in high-risk areas. But California, which has been hard hit by wildfires, recently banned this practice, imposing a one-year moratorium. Looking ahead, regulators must strike a careful balance, ensuring that policies protect vulnerable customers, while preventing insurers themselves from going bankrupt.
Insurers are in the business of accurately pricing risk, but assessing and managing their physical climate risk exposure is getting harder. Climate change has altered the risk equation, so that the past is no longer a reliable indicator of the future. As the frequency and severity of natural disasters has increased, measuring and quantifying future risks has become an incredibly complex task.
Catastrophic loss modeling
In the 1980s, catastrophic loss modeling, also known as ‘cat’ modeling, was developed in the fields of property insurance and the science of natural hazards to estimate the frequency, intensity and potential damage of events such as tropical cyclones, floods and wildfires. The basic structure of cat models takes an event-based/historical approach to modeling (see Table 1). By combining four modules: stochastic, hazard, vulnerability and financial, cat models aim to quantify uncertainties by simulating a set of events and calculating the amount of insured loss each causes.
But events can have complex, varied impacts. As catastrophic events are becoming more unpredictable, frequent and severe, cat models’ reliance on historical data limits their ability to accurately capture future physical climate risks. While good quality data is important, past losses are not always equivalent to future losses. At the outer end of the tail there is no historical data to feed the models, making them less able to explain more extreme events.
Table 1: The methodology of catastrophic loss models
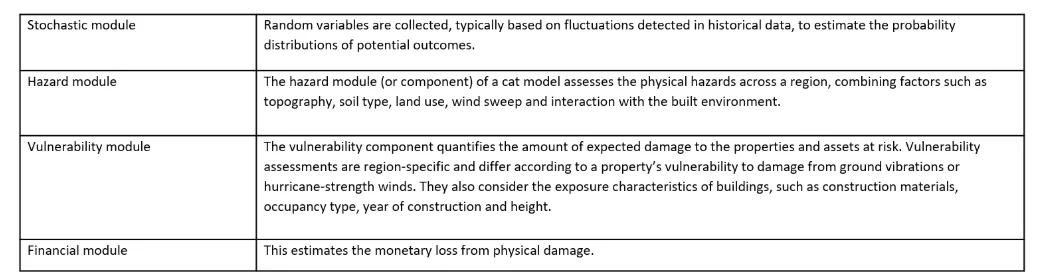
Source: Chartis Research
Computation and data issues
While there is general scientific consensus that climate change is likely to persist, its precise timings, scope and scale are still being debated. As soon as modeling starts, assumptions are made; the reliability of a model also depends on the quantity and quality of its calibration data. To precisely determine when and where climate-related disasters will occur requires large amounts of computational power and data at a more granular level than is currently unavailable. This is especially true for extreme weather events (such as floods, storms, droughts and wildfires) that are periodic and acute. Models are only as useful as the data that goes into them. Inaccurate data could seriously slow efforts to measure and quantify future risks appropriately.
Data quality is also affected by data gaps. In addition to climatic changes, changes in urbanization, building standards and infrastructure are critical factors in attempting to track and create comparable data points to build future scenarios. In addition, impacts of and changes in extreme weather events are uneven across different regions and geographies. In a recent report analyzing the effects of climate change, Goldman Sachs warned of significant risks to the world’s largest cities (such as New York, Tokyo and Lagos), which are especially vulnerable to more frequent storms, storm surges, higher temperatures, and rising sea levels. Climate change can impact weather patterns, which could increase or decrease the correlations between regional risks. And different researchers may measure these weather patterns, such as wind speed, in different ways, creating challenges around the consistency of cat models.
Testing the waters
Despite the difficulty of quantifying physical climate risks, insurers are facing pressure from investors and shareholders to disclose them. Similarly, in an effort to quantify the impact of climate change on financial stability, the Bank of England (BoE) has proposed its 2021 Biennial Exploratory Scenario (BES) exercise for the UK’s largest banks and insurers.
These stress tests will assess firms’ resilience against more frequent severe weather events such as floods and subsidence, and the financial system’s exposure more broadly to climate-related risk. In the most severe scenario, lenders and insurers are likely to be tested against temperature rises as high as 4 degrees Celsius by 2080. These projections are reasonable, because – as the International Energy Agency (IEA) found in its World Energy Outlook 2019 – the expected increase in renewable energy will not be enough to put a ceiling on the energy sector’s emissions before 2040. According to estimates by scientists in the UN’s Emissions Gap Report 2019, the current pledges made by countries under the Paris Agreement would cause temperature rises of about 3.2 degrees Celsius this century.
However, the BoE stress test will not assess companies’ capital adequacy to withstand the impact of climate change on their assets and business models, and the Bank will disclose aggregate results, rather than those for individual firms. For individual insurers, the test aims to improve their risk management by providing a better understanding of the risks facing their business models. At the level of the entire financial system, the tests would illuminate broader risks from second-order effects, unintended consequences, and material disruption to the delivery of financial services.
The tests are part of a crucial agenda to improve firms’ climate readiness, and a way for insurers to demonstrate they have the capabilities to measure and manage climate risk. Not surprisingly, perhaps, insurers that are already engaging with the climate science community to gain a better understanding of the latest data are likely to develop greater resilience to climate-related risks.
But there is an important trade-off: to help firms run the tests within the required timeframe, the BoE has been relatively prescriptive in its approach. Fixed standardized scenarios may limit insurers’ scope to establish their own data analysis and risk management competences for scenarios. And the lack of proven methodologies and data covering the cost of climate risk complicates and potentially undermines the robustness and feasibility of models.
A way forward?
The value of cat models to the insurance industry can be extended by adopting so-called ‘ensemble’ techniques, which can significantly improve the stability and accuracy of models. The most common approach is to run cat models alongside general circulation models (GCMs), also known as ‘earth system’ models. GCMs use mathematical equations to simulate the general movement and circulation of the Earth’s atmosphere, ocean and land surface. GCMs divide the Earth’s surface into a three-dimensional grid of cells, and modelers can create a set of synthetic events, using adjustable parameters from historical data, to determine whether the simulated earth produces consistent and realistic results. The simulated disaster scenarios often follow those outlined by the Intergovernmental Panel on Climate Change (IPCC).
GCMs have considerable potential to provide consistent forecasts of future climate change, especially for larger countries and even continents. Predictive capability is higher for some climate variables – such as temperature – than for others, like precipitation. As most physical processes related to clouds occur on smaller scales, they are harder to model, and their known properties must be averaged over larger scales through ‘parameterization’.** Essentially, a climate model typically has one value for each substance, and by approximating key parameters it provides an average of the process. However, parameterizations can introduce uncertainty into climate models, because narrowing parameterized variables into a single value is a challenge, so the model needs to include an estimate. To determine a model’s accuracy, it must be validated, which is a time-consuming and resource-intensive process, because the model is tested against past analysis using a comprehensive verification process. Also, as with cat models, past data can no longer be used to accurately estimate future events, because those events are becoming more frequent and severe.
Nevertheless, in quantifying climate exposures, a combined GCM and cat model approach would allow for greater flexibility among alternative options, enabling a model to predict more accurately. By using more than one type of model concurrently and combining databases, insurers can plan for a range of potential climate-change impacts. This is the most practical approach, given the long-term time horizon and large number of variables involved.
The bigger picture
More broadly, greater collective action across industries and countries is required to mitigate the impacts of climate change. Nearly 200 countries ratified the 2015 Paris Agreement to limit global warming to well below 2 degrees Celsius, and are making efforts to limit it to 1.5 degrees. But the gap between objectives and implementation remains significant. The Global Carbon Project found that emissions grew by 1.5% in 2017 and by 2.1% in 2018. In 2019, despite a slowdown in growth due largely to an unexpected decline in global coal use in the US and Europe, CO2 from fossil fuels increased by 0.6%.
And the small downturn is nothing to be overly enthusiastic about. Increases through 2020 remain uncertain but likely, because of continued growth in the use of oil and natural gas. The IEA estimates that carbon emissions are on track to rise by 100m tonnes a year for at least another 20 years under existing policy plans. The UN has warned that global emissions must drop by 7.6% a year from now until 2030 to stay beneath the 1.5-degree ceiling and avoid disastrous consequences.
As a result, under current policy plans in many sectors, especially insurance and energy, climate risks are likely to be more complex and severe than anticipated. Low-carbon technologies have been deployed with varying degrees of success, but to attain the drastic cuts in emissions required, more action to displace technologies that emit CO2 is essential, especially in countries where demand for energy is increasing.
While some positive steps have been taken to prioritize climate change on the corporate agenda, more collective action is essential to improve both the readiness of businesses – through enhanced governance, reporting and risk management – and to attain the goals of the Paris Agreement. By analyzing climate risk collaboratively at the system level, the costs of investing in resilience can be quantified and more effectively and fairly distributed. Given how complex climate-change modeling can be, the financial industry as a whole must devote more resources to upskilling its senior managers. Likewise, increased and more productive engagement with the climate science community will be vital to help firms quantify exposure to climate-change risks and the expected losses from increasingly severe events.
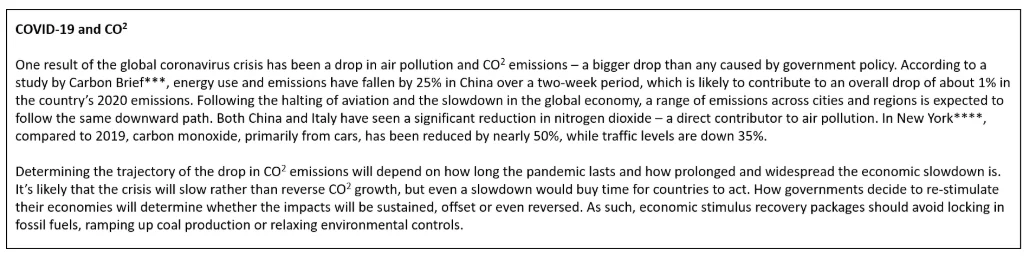
* First-order risks include immediate property damage or bodily injury arising from weather-related events such as flood and storms. Second-order risks are consequential losses, such as a reduction in production or profits. Third-order risks include losses from reduced market share or increased public anger, and are usually not insurable.
** Parameterization techniques replace and simplify processes that are too small-scale or complex to be physically represented in a model.
*** Source can be found here.
**** Source can be found here.
Further reading
- Model Validation Solutions, 2019: Overview and Market Landscape
(2019, Chartis)
- Climate Risk – Special Report 2019
(2019, Risk.net)
- The Economics of Climate
(2019, IMF)
- Catastrophe Modelling and Climate Change
(2014, Lloyd’s)
Points of View are short articles in which members of the Chartis team express their opinions on relevant topics in the risk technology marketplace. Chartis is a trading name of Infopro Digital Services Limited, whose branded publications consist of the opinions of its research analysts and should not be construed as advice.
If you have any comments or queries on Chartis Points of View, you can email the individual author, or email Chartis at info@chartis-research.com.
Only users who have a paid subscription or are part of a corporate subscription are able to print or copy content.
To access these options, along with all other subscription benefits, please contact info@risk.net or view our subscription options here: http://subscriptions.risk.net/subscribe
You are currently unable to print this content. Please contact info@chartis-research.com to find out more.
You are currently unable to copy this content. Please contact info@chartis-research.com to find out more.
Copyright Infopro Digital Limited. All rights reserved.
As outlined in our terms and conditions, https://www.infopro-digital.com/terms-and-conditions/subscriptions/ (point 2.4), printing is limited to a single copy.
If you would like to purchase additional rights please email info@chartis-research.com
Copyright Infopro Digital Limited. All rights reserved.
You may share this content using our article tools. As outlined in our terms and conditions, https://www.infopro-digital.com/terms-and-conditions/subscriptions/ (clause 2.4), an Authorised User may only make one copy of the materials for their own personal use. You must also comply with the restrictions in clause 2.5.
If you would like to purchase additional rights please email info@chartis-research.com